Learning to Factorize and Relight a City
ECCV 2020
UC Berkeley
Humen AI
UC Berkeley
Abstract
We propose a learning-based framework for disentangling outdoor scenes into temporally-varying illumination and permanent scene factors. Inspired by the classic intrinsic image decomposition, our learning signal builds upon two insights: 1) combining the disentangled factors should reconstruct the original image, and 2) the permanent factors should stay constant across multiple temporal samples of the same scene. To facilitate training, we assemble a city-scale dataset of outdoor timelapse imagery from Google Street View, where the same locations are captured repeatedly through time. This data represents an unprecedented scale of spatio-temporal outdoor imagery. We show that our learned disentangled factors can be used to manipulate novel images in realistic ways, such as changing lighting effects and scene geometry.
Results
Paper
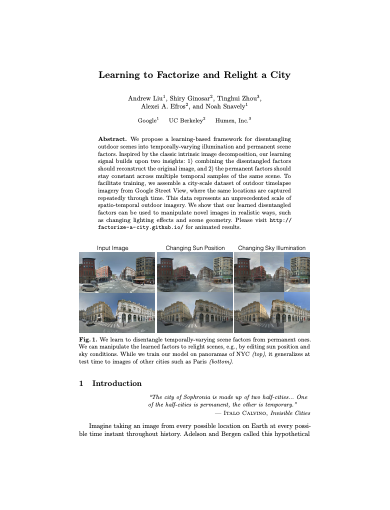
A. Liu, S. Ginosar, T. Zhou, A. A. Efros, N. Snavely
In ECCV, 2020
[Bibtex] [arXiv] [Supplementary]
Additional Results
We present additional generated results. Despite training on scenes from New York City, we can relight scenes from entirely new cities and even continents. Other cities coming soon!